Stay updated
News & Insightsexampleexample bboxesexample bboxes2example chromatic aberrationexample d4example documentsexample domain adaptationexample gridshuffleexample hfhubexample kaggle saltexample keypointsexample mosaicexample multi targetexample OverlayElementsexample textimageexample weather transformsexample xymaskingmigrating from torchvision to albumentationspytorch classificationpytorch semantic segmentationreplayserializationshowcase
Mosaic Transform Example 🔗
This notebook demonstrates the Mosaic
augmentation from Albumentations.
Mosaic
combines multiple images and their corresponding annotations (masks, bounding boxes, keypoints) into a single larger image, arranging them in a grid.
Key Features Illustrated:
- Combining Multiple Inputs: Shows how
Mosaic
uses a primary image and additional images provided via metadata. - Handling Annotations: Demonstrates the transformation of masks, bounding boxes, and keypoints alongside the images.
- Label Handling: Explains how labels for bounding boxes and keypoints are managed, especially when combining items with potentially different label sets.
- Geometric Adjustments: Illustrates how images/annotations are cropped, resized, and placed onto the final mosaic canvas.
import cv2
import matplotlib.pyplot as plt
import numpy as np
# Helper functions for visualization
def visualize_image_mask(image, mask, title="Image and Mask"):
fig, ax = plt.subplots(1, 2, figsize=(10, 5))
image = image.copy()
mask = mask.copy()
ax[0].imshow(image)
ax[0].set_title("Image")
ax[0].axis("off")
# Ensure mask is 2D for grayscale display
if mask.ndim == 3 and mask.shape[2] == 1:
mask_display = mask[:, :, 0]
else:
mask_display = mask
ax[1].imshow(mask_display, cmap="gray")
ax[1].set_title("Mask")
ax[1].axis("off")
fig.suptitle(title)
plt.tight_layout()
plt.show()
def visualize_bbox(img, bboxes, labels=None, title="Image with Bounding Boxes"):
img_copy = img.copy()
# Convert to RGB for display if in BGR
# Use a colormap for different classes
unique_labels = list(set(labels)) if labels else []
colors = plt.cm.viridis(np.linspace(0, 1, max(len(unique_labels), 1)))
for i, bbox in enumerate(bboxes):
x_min, y_min, x_max, y_max = map(int, bbox[:4])
# Get color based on label
if labels is not None and i < len(labels):
label_text = str(labels[i])
# Find index of this label in unique_labels
color_idx = unique_labels.index(label_text) if label_text in unique_labels else 0
color = tuple(int(c * 255) for c in colors[color_idx][:3]) # RGBA to BGR
else:
label_text = ""
color = (0, 255, 0) # Default green
cv2.rectangle(img_copy, (x_min, y_min), (x_max, y_max), color, 2)
if label_text:
cv2.putText(img_copy, label_text, (x_min, y_min - 10), cv2.FONT_HERSHEY_SIMPLEX, 0.5, color, 2)
plt.figure(figsize=(10, 10))
plt.imshow(img_copy)
plt.title(title)
plt.axis("off")
plt.show()
def visualize_keypoints(img, keypoints, labels=None, title="Image with Keypoints"):
img_copy = img.copy()
# Use a colormap for different keypoint labels
unique_labels = list(set(labels)) if labels else []
colors = plt.cm.viridis(np.linspace(0, 1, max(len(unique_labels), 1)))
for i, kp in enumerate(keypoints):
x, y = map(int, kp[:2])
# Get color based on label
if labels is not None and i < len(labels):
label_text = str(labels[i])
# Find index of this label in unique_labels
color_idx = unique_labels.index(label_text) if label_text in unique_labels else 0
color = tuple(int(c * 255) for c in colors[color_idx][:3]) # RGBA to BGR
else:
label_text = ""
color = (0, 0, 255) # Default red
# Draw circle for keypoint
cv2.circle(img_copy, (x, y), 5, color, -1)
plt.figure(figsize=(10, 10))
plt.imshow(img_copy)
plt.title(title)
plt.axis("off")
plt.show()
import json
import os
import albumentations as A
# Function to parse LabelMe JSON format
def parse_labelme_json(json_path):
"""Parse LabelMe JSON annotations to extract bounding boxes, masks, and keypoints."""
with open(json_path) as f:
data = json.load(f)
image_path = os.path.join(os.path.dirname(json_path), data["imagePath"])
# Read as RGB directly
image = cv2.imread(image_path, cv2.IMREAD_COLOR_RGB)
height, width = image.shape[:2]
# Initialize outputs
bboxes = []
keypoints = []
mask = np.zeros((height, width), dtype=np.uint8)
bbox_labels = []
keypoint_labels = []
for shape in data["shapes"]:
label = shape["label"]
shape_type = shape["shape_type"]
points = np.array(shape["points"])
if shape_type == "rectangle":
# Rectangle is specified by two points: top-left and bottom-right
x_min, y_min = points[0]
x_max, y_max = points[1]
# Add to bboxes in [x_min, y_min, x_max, y_max] format
bboxes.append([x_min, y_min, x_max, y_max])
bbox_labels.append(label)
elif shape_type == "polygon":
# Convert polygon to mask - don't add as a bounding box
points = points.astype(np.int32)
cv2.fillPoly(mask, [points], 255) # Fill with 255 (white)
elif shape_type == "point":
# Handle keypoints
x, y = points[0]
keypoints.append([x, y, 2]) # 2 means visible keypoint
keypoint_labels.append(label)
# Convert to format expected by albumentations
if bboxes:
bboxes = np.array(bboxes, dtype=np.float32)
else:
bboxes = np.zeros((0, 4), dtype=np.float32)
if keypoints:
keypoints = np.array(keypoints, dtype=np.float32)
else:
keypoints = np.zeros((0, 3), dtype=np.float32)
return {
"image": image,
"bboxes": bboxes,
"keypoints": keypoints,
"mask": mask,
"bbox_labels": bbox_labels,
"keypoint_labels": keypoint_labels,
"image_path": image_path,
}
# Visualization helper function
def visualize(image, bboxes=None, keypoints=None, mask=None, bbox_labels=None, keypoint_labels=None):
"""Visualize an image with its bounding boxes, keypoints, and masks."""
plt.figure(figsize=(12, 10))
plt.imshow(image)
if mask is not None and np.any(mask):
# Display mask as a semi-transparent overlay
mask_img = np.zeros_like(image)
mask_img[:, :, 0] = mask # Set red channel
plt.imshow(mask_img, alpha=0.3)
if bboxes is not None and len(bboxes) > 0:
for i, bbox in enumerate(bboxes):
x_min, y_min, x_max, y_max = bbox
rect = plt.Rectangle((x_min, y_min), x_max - x_min, y_max - y_min, fill=False, edgecolor="red", linewidth=2)
plt.gca().add_patch(rect)
# Add label if available
if bbox_labels is not None and i < len(bbox_labels):
plt.text(x_min, y_min - 5, bbox_labels[i], color="red", fontsize=12, backgroundcolor="white")
if keypoints is not None and len(keypoints) > 0:
for i, kp in enumerate(keypoints):
x, y, v = kp
if v > 0: # if keypoint is visible
plt.scatter(x, y, s=50, c="blue", marker="o")
plt.axis("off")
plt.tight_layout()
plt.show()
# Load example data
print("Loading and visualizing the first few images...")
image_dir = "images/mosaic" # Changed from notebooks/images/mosaic
json_files = [f for f in os.listdir(image_dir) if f.endswith(".json")]
print(json_files)
examples = []
for json_file in json_files: # Load first three as examples
json_path = os.path.join(image_dir, json_file)
example = parse_labelme_json(json_path)
examples.append(example)
print(f"Visualizing {json_file}...")
visualize(
example["image"],
example["bboxes"],
example["keypoints"],
example["mask"],
example["bbox_labels"],
example["keypoint_labels"],
)
print(f"Found {len(example['bboxes'])} bounding boxes and {len(example['keypoints'])} keypoints")
print("-" * 50)
Loading and visualizing the first few images... ['billy.json', 'skull.json', 'road.json', 'spectrogram.json', 'driving.json'] Visualizing billy.json...
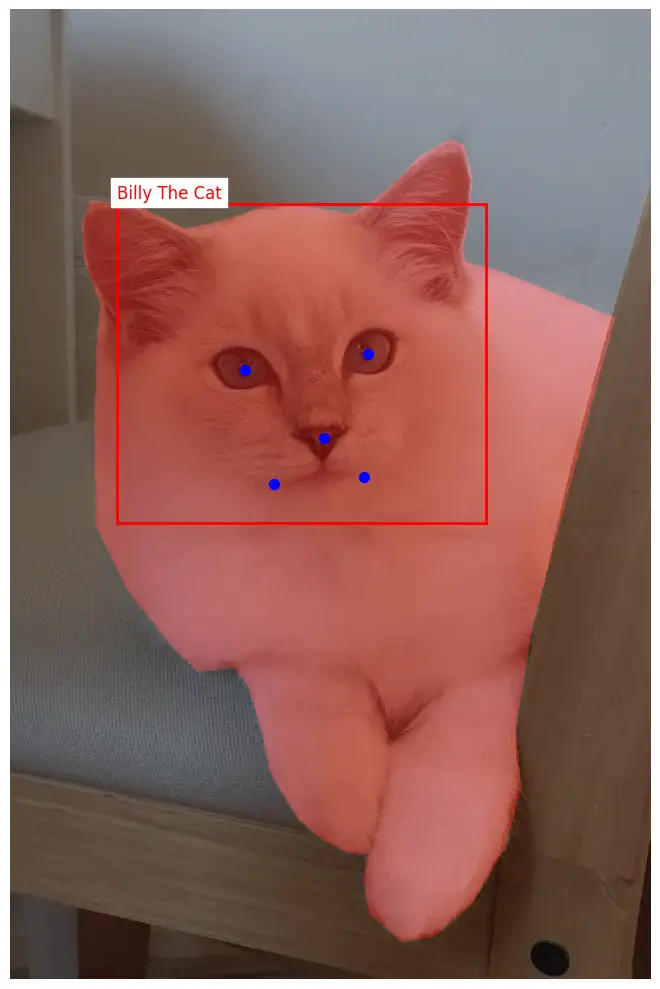
Found 1 bounding boxes and 5 keypoints 🔗
Visualizing skull.json...
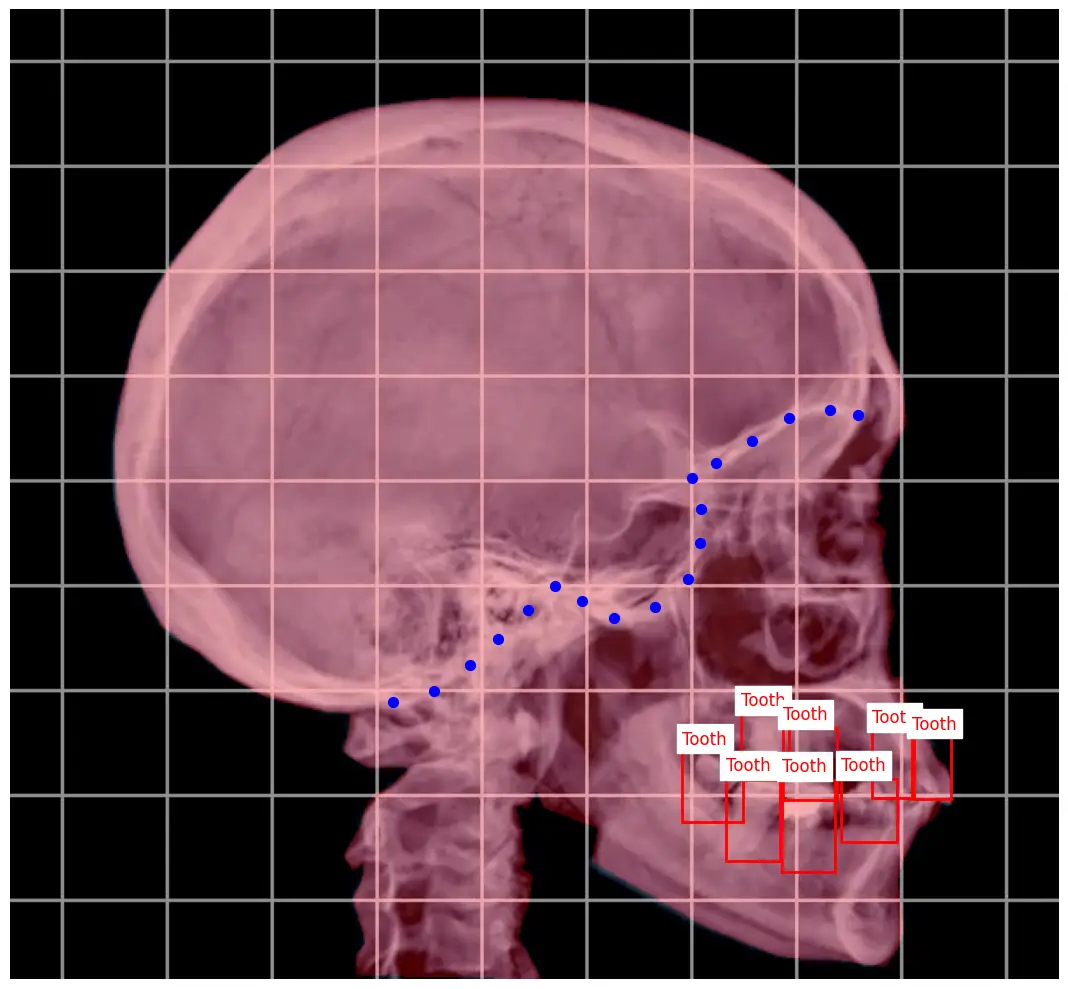
Found 8 bounding boxes and 18 keypoints 🔗
Visualizing road.json...
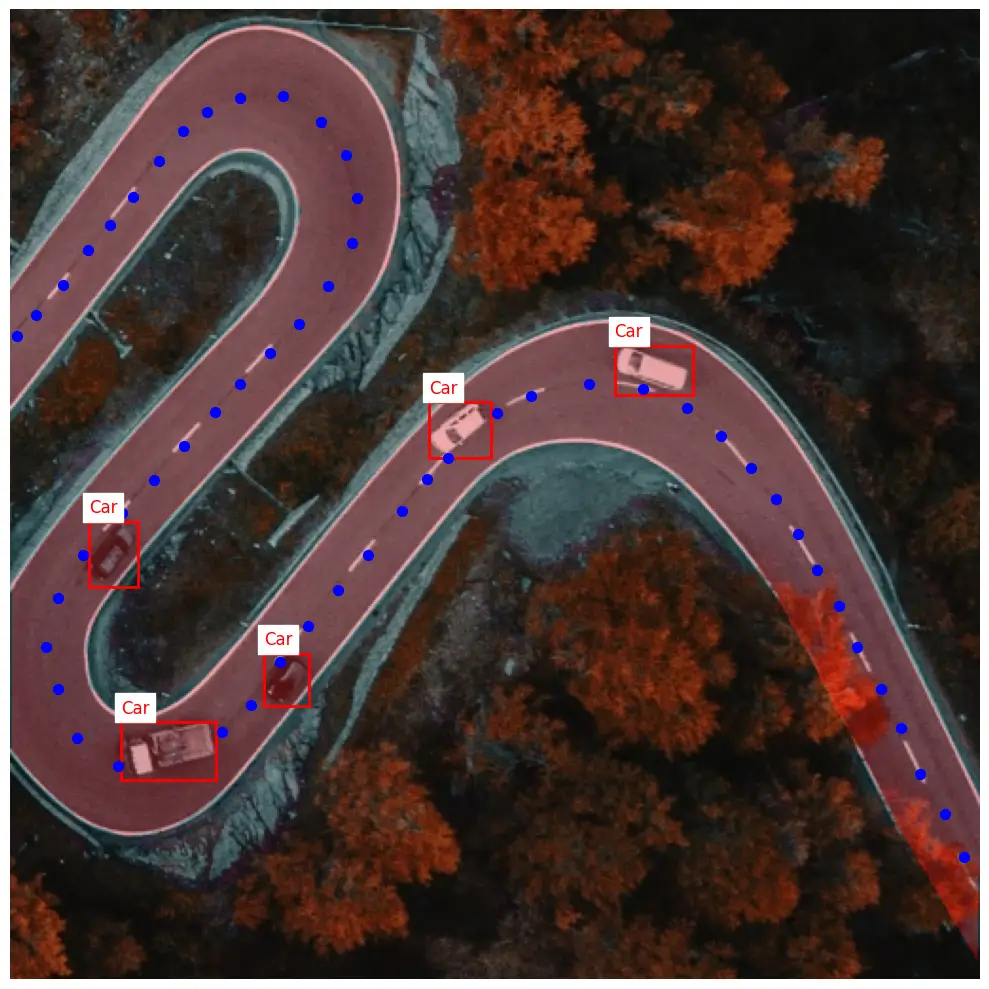
Found 5 bounding boxes and 55 keypoints 🔗
Visualizing spectrogram.json...
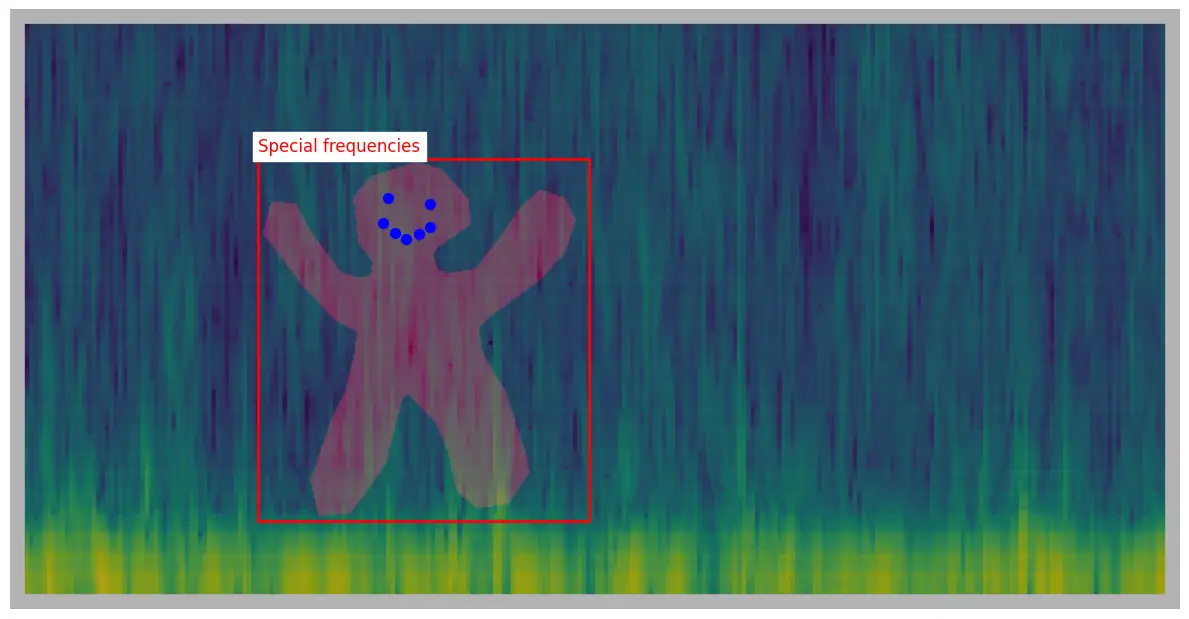
Found 1 bounding boxes and 7 keypoints 🔗
Visualizing driving.json...
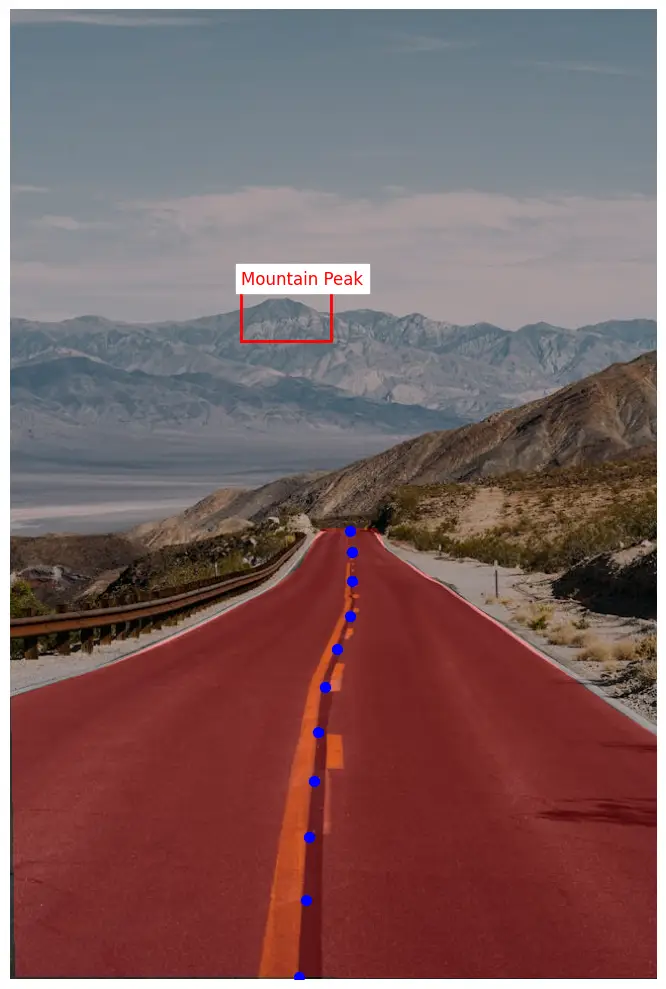
Found 1 bounding boxes and 11 keypoints 🔗
# Now let's define the Mosaic transform and apply it
def get_mosaic_transform(grid_yx=(3, 3), target_size=(1024, 1024)):
"""Define a Mosaic transform."""
transform = A.Compose(
[
A.Mosaic(
grid_yx=grid_yx,
cell_shape=(512, 512),
fit_mode="contain",
target_size=target_size,
metadata_key="mosaic_metadata",
p=1,
),
],
bbox_params=A.BboxParams(format="pascal_voc", label_fields=["bbox_labels"]),
keypoint_params=A.KeypointParams(format="xy", label_fields=["keypoint_labels"]),
p=1,
)
return transform
# Prepare the input dictionary for the transform pipeline
# Extract the primary image and its annotations
primary_example = examples[0]
primary_data = {
"image": primary_example["image"],
"bboxes": primary_example["bboxes"],
"keypoints": primary_example["keypoints"],
"mask": primary_example["mask"],
"bbox_labels": primary_example["bbox_labels"],
"keypoint_labels": primary_example["keypoint_labels"],
# Provide additional images as metadata for the Mosaic transform
"mosaic_metadata": examples[1:],
}
print(len(examples))
# Apply the transform
mosaic_transform = get_mosaic_transform()
result = mosaic_transform(**primary_data)
# Visualize the result
# plt.figure(figsize=(14, 12))
plt.imshow(result["image"])
# Draw bounding boxes
for i, bbox in enumerate(result["bboxes"]):
x_min, y_min, x_max, y_max = bbox[0], bbox[1], bbox[2], bbox[3]
rect = plt.Rectangle((x_min, y_min), x_max - x_min, y_max - y_min, fill=False, edgecolor="red", linewidth=2)
plt.gca().add_patch(rect)
# Add label if available
if "bbox_labels" in result and i < len(result["bbox_labels"]):
plt.text(x_min, y_min - 5, result["bbox_labels"][i], color="red", fontsize=10, backgroundcolor="white")
# Draw keypoints
if "keypoints" in result and len(result["keypoints"]) > 0:
for i, kp in enumerate(result["keypoints"]):
x, y = kp[0], kp[1]
plt.scatter(x, y, s=30, c="blue", marker="o")
plt.title("Mosaic Transform Result")
plt.axis("off")
plt.tight_layout()
plt.show()
print(f"After Mosaic: {len(result['bboxes'])} bounding boxes and {len(result['keypoints'])} keypoints")
5
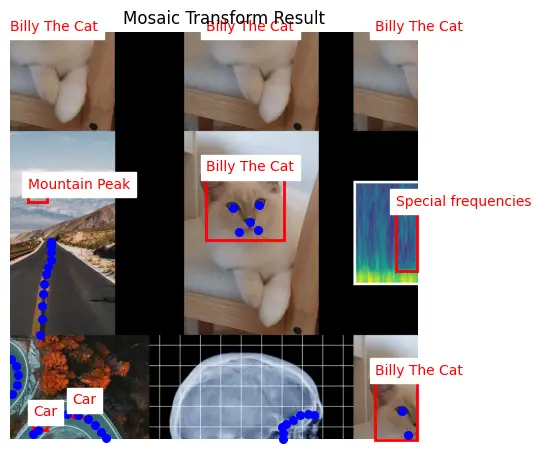
After Mosaic: 9 bounding boxes and 41 keypoints