Stay updated
News & Insightsexampleexample bboxesexample bboxes2example chromatic aberrationexample d4example documentsexample domain adaptationexample gridshuffleexample hfhubexample kaggle saltexample keypointsexample mosaicexample multi targetexample OverlayElementsexample textimageexample weather transformsexample xymaskingmigrating from torchvision to albumentationspytorch classificationpytorch semantic segmentationreplayserializationshowcase
%matplotlib inline
RandomGridShuffle 🔗
This transformation divides the image into a grid and then permutes these grid cells based on a random mapping.
It could be useful when only micro features are important for the model, and memorizing the global structure could be harmful.
For example:
- Identifying the type of cell phone used to take a picture based on micro artifacts generated by phone post-processing algorithms, rather than the semantic features of the photo. See more at https://ieeexplore.ieee.org/abstract/document/8622031
- Identifying stress, glucose, hydration levels based on skin images.
import albumentations as A
import cv2
from matplotlib import pyplot as plt
/opt/homebrew/Caskroom/miniconda/base/envs/albumentations_examples/lib/python3.9/site-packages/tqdm/auto.py:21: TqdmWarning: IProgress not found. Please update jupyter and ipywidgets. See https://ipywidgets.readthedocs.io/en/stable/user_install.html from .autonotebook import tqdm as notebook_tqdm
import json
KEYPOINT_COLOR = (0, 255, 0)
def vis_keypoints(image, keypoints, color=KEYPOINT_COLOR, diameter=3):
image = image.copy()
for x, y in keypoints:
cv2.circle(image, (int(x), int(y)), diameter, color, -1)
return image
def visualize(image, mask, keypoints):
# Create a copy of the image to draw on
img = image.copy()
# Apply keypoints if provided
if keypoints:
img = vis_keypoints(img, keypoints)
# Setup plot
fig, ax = plt.subplots(1, 2, figsize=(10, 5))
# Show the image with annotations
ax[0].imshow(img)
ax[0].axis("off")
# Show the mask
ax[1].imshow(mask, cmap="gray")
ax[1].axis("off")
plt.tight_layout()
plt.show()
with open("../data/road_labels.json") as f:
labels = json.load(f)
keypoints = labels["keypoints"]
image = cv2.imread("../data/road.jpeg", cv2.IMREAD_COLOR_RGB)
mask = cv2.imread("../data/road.png", 0)
visualize(image, mask, keypoints)
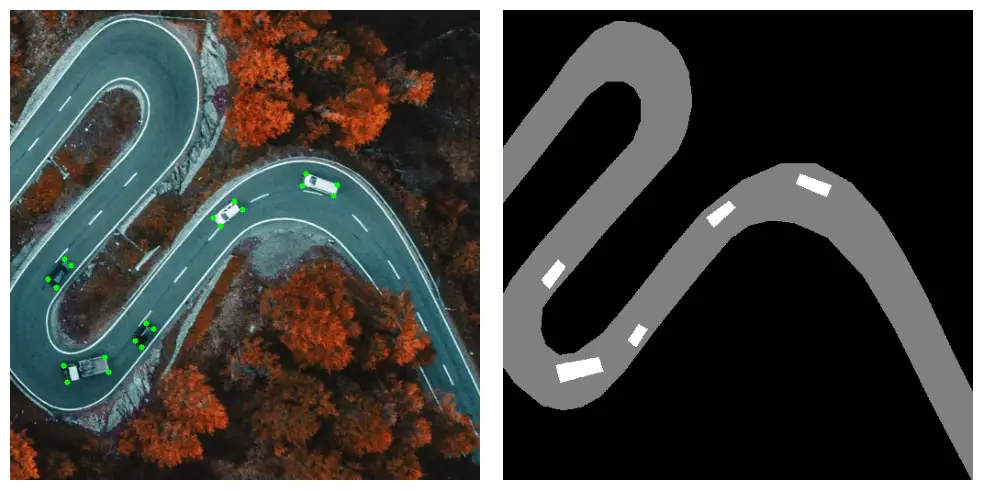
transform = A.Compose([A.RandomGridShuffle(grid=(2, 2), p=1)], keypoint_params=A.KeypointParams(format="xy"))
transformed = transform(image=image, keypoints=keypoints, mask=mask)
visualize(transformed["image"], transformed["mask"], transformed["keypoints"])
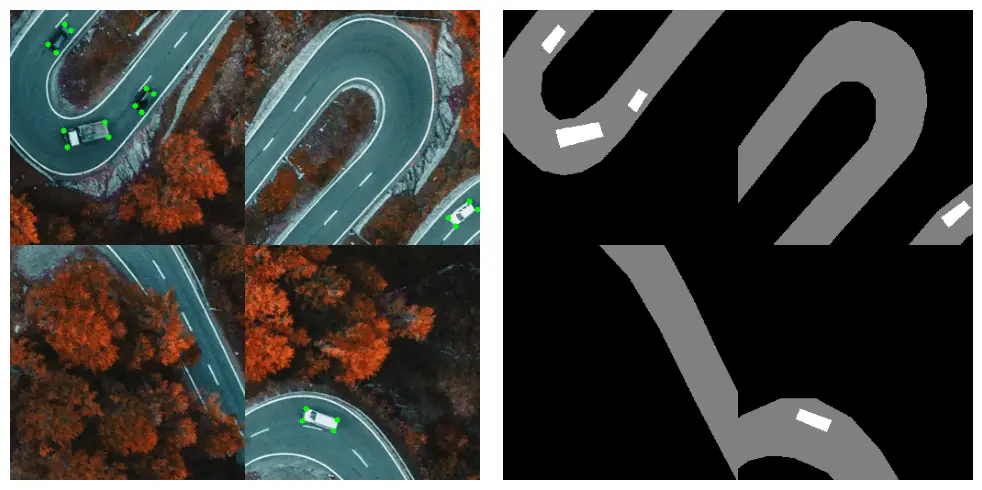
transform = A.Compose(
[A.RandomGridShuffle(grid=(3, 3), p=1)],
keypoint_params=A.KeypointParams(format="xy"),
seed=137,
strict=True,
)
transformed = transform(image=image, keypoints=keypoints, mask=mask)
visualize(transformed["image"], transformed["mask"], transformed["keypoints"])
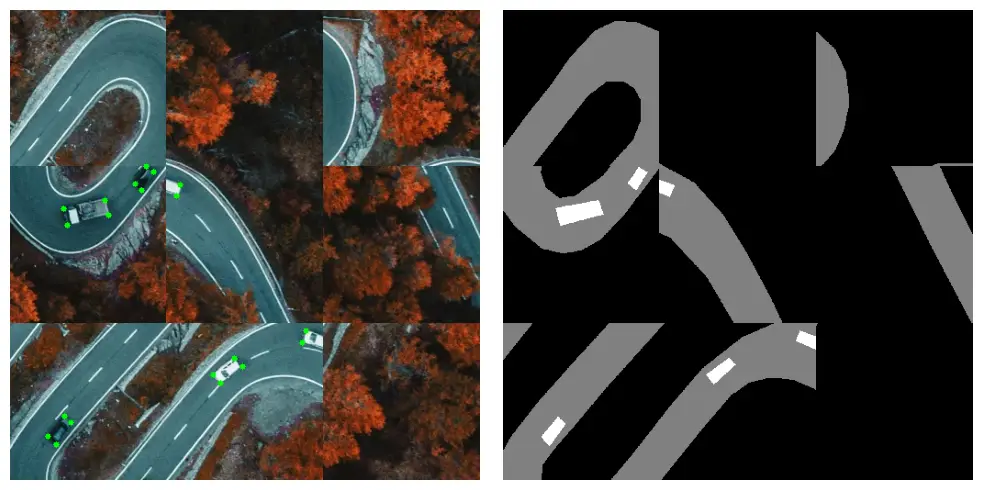
transform = A.Compose(
[A.RandomGridShuffle(grid=(5, 7), p=1)],
keypoint_params=A.KeypointParams(format="xy"),
seed=137,
strict=True,
)
transformed = transform(image=image, keypoints=keypoints, mask=mask)
visualize(transformed["image"], transformed["mask"], transformed["keypoints"])
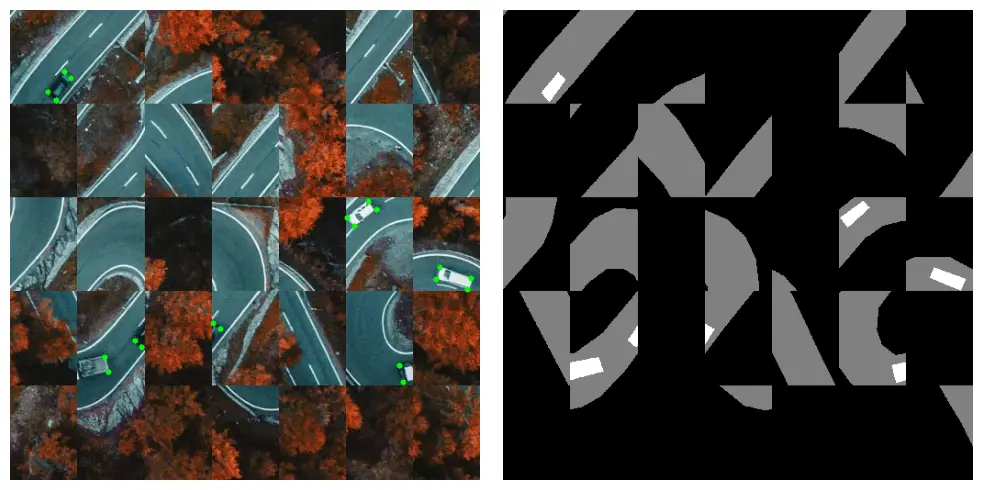